Making Better Use of Big Data in Marketing
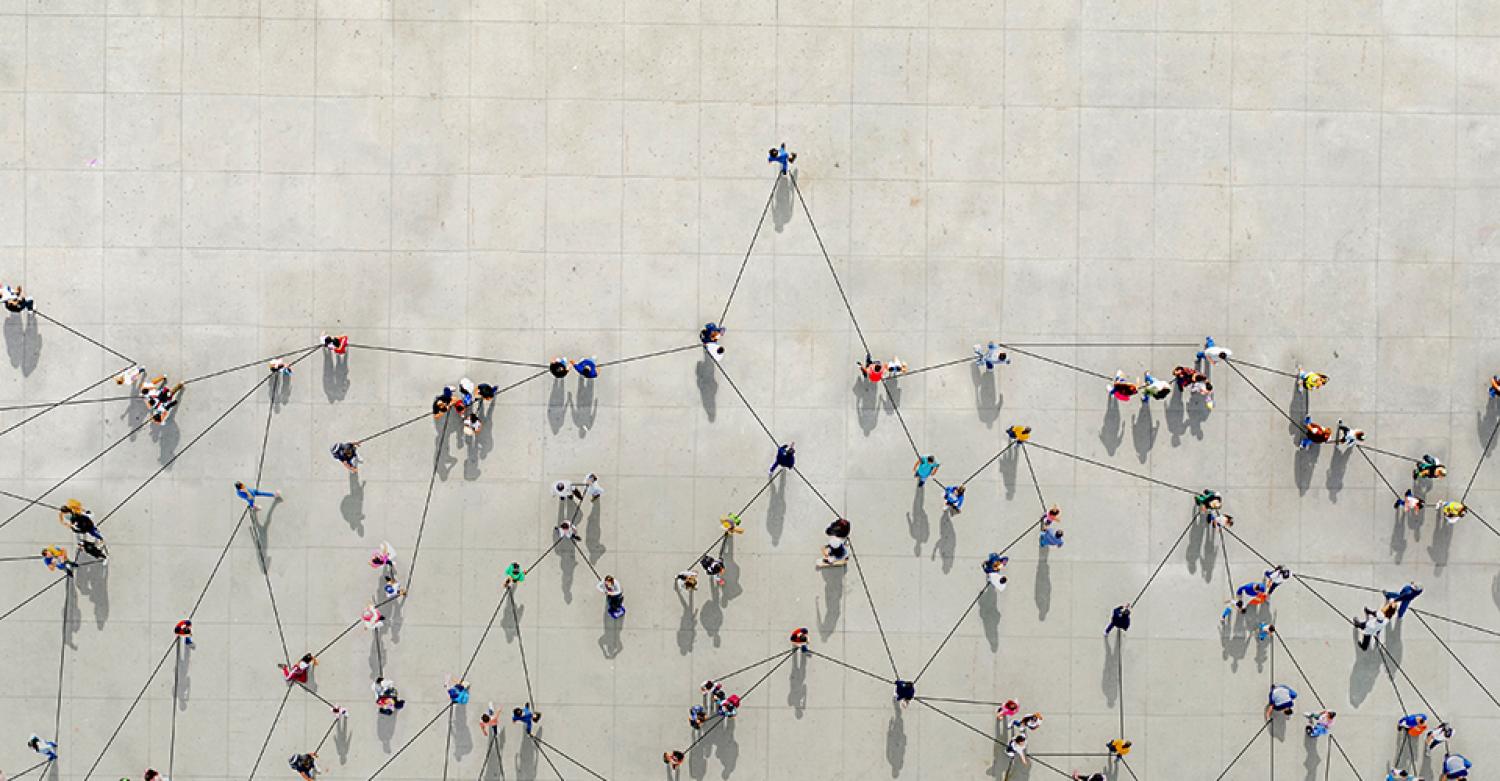
Leeds professor earns Kahle award for algorithm aimed at improved targeting.
A paper aimed at helping businesses better target consumers through personalized advertising of curated products is this year’s winner of the Kahle Family Research Award at Leeds.
Rico Bumbaca, an assistant professor of marketing at Leeds, is the winner of this distinguished honor, presented to one exceptional faculty research paper published in an elite journal.
“I’m still very new to Leeds, I’m only an assistant professor,” said Prof. Bumbaca, who earned his PhD from the University of California Irvine. “I don’t consider myself to be in the same league as my colleagues, who are brilliant and much more seasoned. I am stunned and humbled by this honor.”
He may be new, but Prof. Bumbaca’s work merits distinction for its potential applications in industry. Alongside researchers from the University of Chicago’s Booth School of Business and the Anderson School of Management at UCLA, he developed an algorithm that quickly scales large data sets to generate highly accurate projections of customers’ wants and desires.
This is a crucial unmet need, as for all the data potential customers are generating, many marketing departments remain unsophisticated when it comes to identifying the right message for the right customer.
Bumbaca and his team describe how this method works in their new paper, “Scalable Target Marketing: Distributed Markov Chain Monte Carlo for Bayesian Hierarchical Models,” which was published in the Journal of Marketing Research in October.
“The method takes advantage of supercomputers by breaking up the data into smaller chunks, processing each chunk in parallel and combining the results to provide very precise estimates of a consumer’s preferences,” Prof. Bumbaca said.
This information about consumers’ preferences can then be used by firms to more accurately target their messaging and increase the likelihood of consumers’ responses to their advertising.
“The key takeaway is that firms can now provide a win-win for their target customers,”
says Bumbaca.
“Customers win by having fewer annoying messages they need to process from firms, and the messages they do receive are spot-on in terms of meeting their needs,” he said. “Firms win by increasing the efficiency of their marketing efforts at a reduced cost, earning larger returns on their smaller marketing budgets.”
The team applied the method for a charitable organization that wants to more efficiently target potential donors. Using their algorithm, they predicted an increase in $1.6 million to $4.2 million in incremental donations per campaign, more than the amount of donations using a traditional statistical method.
Prof. Bumbaca is already working on other areas related to this research stream. He’s currently investigating a method to calculate the distribution of unique preferences related to a product or service. This is different from target marketing, when you want to know how a particular customer will react to a particular message or product, based on the data she’s generated.
“When you’re looking at a new product line, it’s easy to come up with the two or three biggest segments of your market,” he said. “But with a larger sample size, you may see two or three niche areas you didn’t consider,” opening up potential new markets or applications for a product manager.
Read the abstract and full paper here.