Explainable Artificial Intelligence: Can We Hold Machines Accountable? A Q&A with Professors Surden and Kaminski
Harry Surden and Margot Kaminski, associate professors at the University of Colorado Law School, are leaders in exploring the future of AI and how technologies using computer-based decision making offer major prospects for breakthroughs in the law—and how those decisions are regulated.
They organized a May 3 conference at Colorado Law titled "Explainable Artificial Intelligence: Can We Hold Machines Accountable?" The conference was hosted by the law school’s Silicon Flatirons Center, of which Surden serves as interim executive director and Kaminski as faculty director for its privacy initiative.
We sat down with Surden and Kaminski to get their take on explainable AI and how humans can help guide computers to fulfill their intended purpose: to serve us well.
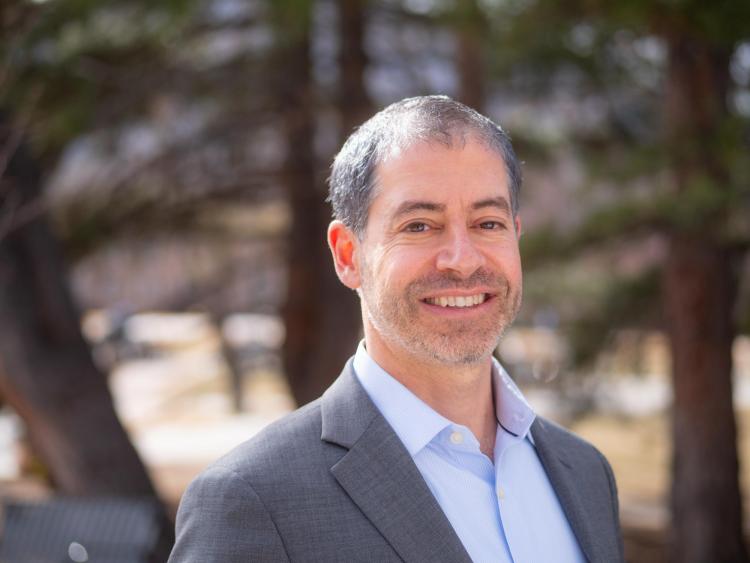
Harry Surden
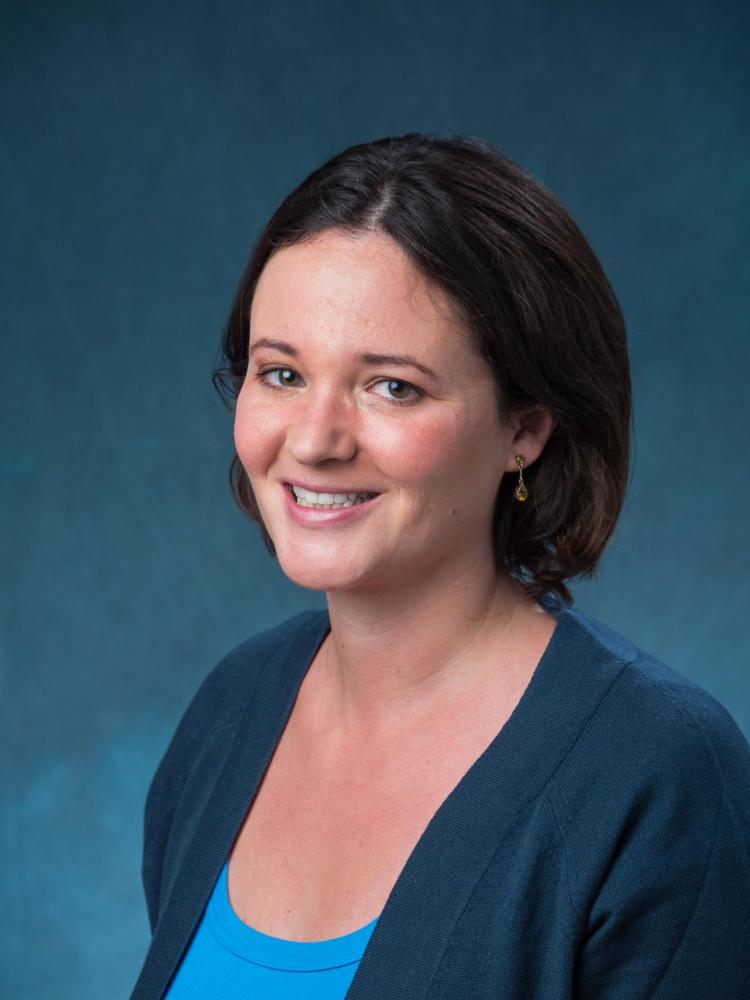
Margot Kaminski
Let’s begin with a definition. What is "explainable" AI?
Kaminski: Explainable AI is AI that provides an explanation of why or how it arrives at a decision/output. What this means, though, depends on whether you ask a lawyer or a computer scientist. This discrepancy is part of what inspired this conference. A lawyer may be interested in different kinds of explanation than a computer scientist, like an explanation that provides insights into whether a decision is justified, whether it is legal, or allows a person to challenge that decision in some way.
What problem is AI explainability trying to solve?
Kaminski: What problem you’re trying to address with explanations can really influence how valuable you think they are, or what form you think they should take. For example, some people focus on the instrumental values of explanations: catching and fixing error or bias or discrimination. Others focus on the role of explanations in preserving human dignity, providing people with the ability to push back against automated decisions and maintain autonomy of some kind. We expect a healthy debate over this at the conference.
Surden: There are certain core legal values—justice, fairness, equality in treatment, due process. To the extent that AI is being used in legal determinations or decisions (e.g., criminal sentencing), there is some sense that legal norms such as justifying government decisions or providing rational or reasonable explanations should be part of that process. One line of thinking is that having AI systems provide explanations might help foster those norms where they are absent today in AI-influenced government determinations.
Legal scholars note that "black box" decision making raises problems of fairness, legitimacy, and error. Why is this concerning to lawyers, governments, policymakers, and others who may be implementing AI in their business practices?
Kaminski: AI decision making is being deployed across the economy and in the government, in areas from hiring and firing to benefits determinations. On the one hand, this can be a good thing: adding statistical analysis into public policy decisions isn’t inherently bad, and can replace human bias. On the other hand, though, there is the real problem of "automation bias," which indicates that humans trust decisions made by machines more than they trust decisions made by other humans. When people use AI to facilitate decisions or make decisions, they’re relying on a tool constructed by other humans. Often they don’t have the technical capacity, or the practical capacity, to determine whether they should be relying on those tools in the first place.
Surden: Part of the legitimacy of the legal system depends upon people believing that they are being fairly, and equally treated, and that government decisions are happening for justifiable reasons. To the extent that AI is being used in government decision making, but remains opaque or inscrutable, this may undermine trust in the legal system and/or government.
Judges increasingly rely on AI systems when making bail or sentencing decisions for criminal defendants, as Professor Surden describes in this podcast. What potential issues, such as racial bias, does this raise? More broadly, how do we avoid feeding biased data to our machine learning systems?
Surden: One of the problems is that the term "bias" itself has many different meanings in different contexts. For example, in computer science and engineering, "bias" is often used as a technical term that often means something akin to "noise" or "skew" in data. But it doesn’t have any sociological or societal meaning in that usage. By contrast, in sociological contexts and in everyday use, "bias" often has a meaning that connotes improper discrimination or treatment of historically oppressed minority groups. There are other, more nuanced meanings as well of bias. While many of these variants of "bias" can exist in AI systems and data, one problem is simply identifying which variants we are talking about or concerned with in any given conversation. Another major issue is that there are many different ways to measure whether data or AI systems are "biased" in improper ways against particular societal groups. It is contested what is the appropriate approach to reduce harm, and what is the "fairest", and this needs to be part of a larger social dialog.
In a recent article, Professor Kaminski points out that the decisions made by machine-learning algorithms, which are used to make significant decisions about individuals from credit determination to hiring and firing, remain largely unregulated under U.S. law. What might effective regulation look like?
Kaminski: To effectively regulate AI, we have to figure out why we want to regulate it. What’s the problem we’re trying to solve? Senators Wyden and Booker just proposed legislation in the United States that would require companies to perform "Algorithmic Impact Assessments" and do risk mitigation around AI bias. That’s great if your only concern is instrumental—fixing bias, but not addressing human dignitary or justificatory concerns—and if you trust that agency enforcement is strong enough that companies will self-regulate without individual challenges. My answer in a nutshell to this is question is: we probably need to do both. We need both a regulatory, systemwide, ex-ante approach to AI biases, and some form of individual transparency or even contestability, to let affected individuals push back when appropriate.
Some claim that transparency rarely comes for free and that there are often tradeoffs between AI’s "intelligence" and transparency. Does AI need to be explainable to be ethical?
Surden: I think that explainability is just one avenue that scholars are pursuing to help address some of the ethical issues raised by the use of AI. I think there are several things we don’t know at this point. First, we don’t know, as a technical matter, whether we will even be able to have AI systems produce explanations that are useful and satisfactory in the context of current law. In the current state of the art, many AI "explanations" are really just dry technical expositions of data and algorithmic structures, and not the kind of justificatory narratives that many people imagine when they hear the term "explanation." So the first issue is whether suitable "explanations" are even achievable as a technological matter in the short term. The longer term questions is—even if AI "explanations" are technically achievable, I don’t think we know to what extent they will even usually solve or address some of the ethical issues that we see today in AI’s public use. It may turn out that we produce useful explanations, but that the “explanation” issue was just a minor problem compared to larger societal issues surrounding AI. Improving "explanation" is just a hypothesis that many scholars are exploring.