PhD student Mitchell Fulton won international medical imaging challenge
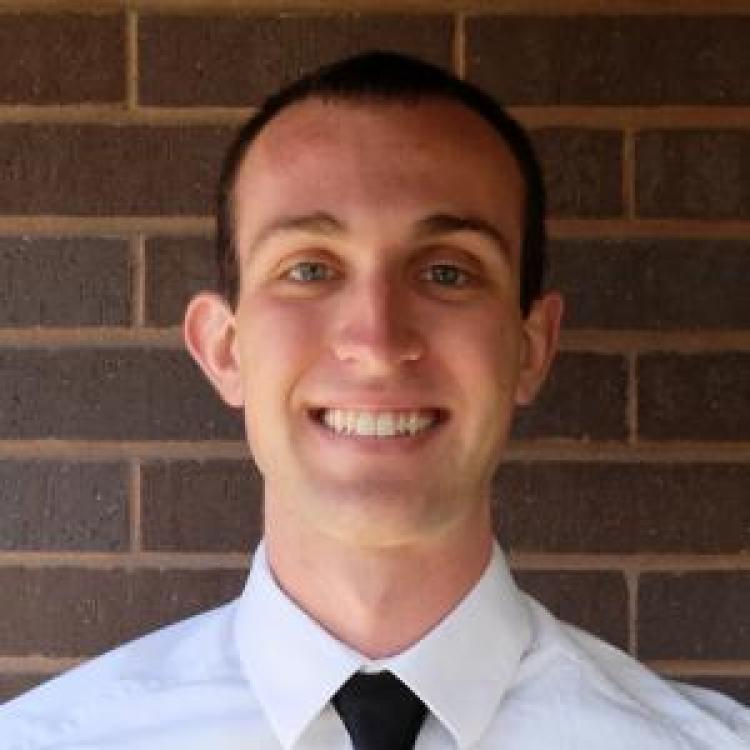
Mitchell Fulton, Mechanical Engineering PhD student
Department of Mechanical Engineering PhD student Mitchell Fulton has won first place in an international medical imaging challenge for a paper detailing his novel method to autonomously identify regions of the heart using MRI scan images.
The Multi-Disease, Multi-View and Multi-Center Right Ventricular Segmentation in Cardiac MRI, or M&Ms-2 Challenge, requires participants to use machine-learning techniques to segment the right ventricle of the heart. The long-term applications of the challenge could help health professionals accurately diagnose cardiac disease.
100 people from around the world participated in the challenge this year. Out of 15 finalists, Fulton won ‘Best Performing Method’ for his approach. The challenge is part of the annual Medical Image Computing and Computer Assisted Intervention Conference (MICCAI).
“It is great to see Mitch show the value of his novel approach in the open competition,” said Fulton’s advisor, Professor Mark Rentschler. “I am very proud of Mitch’s efforts that have led to winning this MICCAI competition, but at the same time, I am very interested in his next research step as he pushes to finish his PhD. I think is best work is yet to come.”
Fulton’s award-winning paper is titled “Deformable Bayesian Convolutional Networks for Disease-Robust Cardiac MRI Segmentation.” It will be published in Springer’s Lecture Notes in Computer Science (LNCS) as part of the Statistical Atlases and Computational Modelling of the Heart (STACOM) 2021 proceedings.
After winning first place in the challenge, Fulton shared more details about his award-winning paper and plans for the future.
Describe the method that you developed to autonomously identify regions of the heart.
We created and used a novel technique called deformable Bayesian convolutional networks. By replacing only one layer of an existing network structure, we increase its generalizability across different heart diseases.
We explored the effects of both training time and network size on generalizability for both 2D long-axis and 3D short-axis cardiac MRIs. We found that the optimum training and network configuration is dependent both on the dataset size and task. We then entered the final test set of the competition to achieve competitive results.
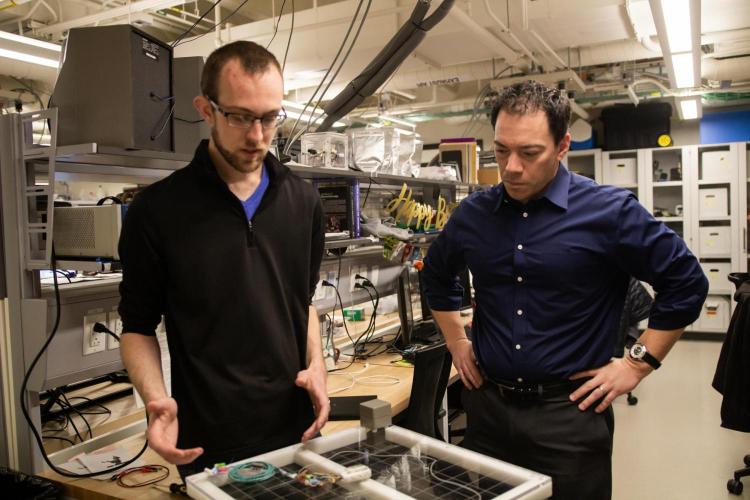
Mitchell Fulton and Professor Mark Rentschler working in Rentschler's Advanced Medical Technologies Laboratory (AMTL).
Was there a particular aspect of this work that was difficult to complete?
Domain generalization problems are difficult in general because you have to balance the ability to perform well on unseen domains with accuracy within the domains you have already seen. Most networks look to do this by adding noise into the training procedure to make the learned features robust, but we wanted to learn the robust features within the structure of the network itself.
We also wanted to apply our technique to medical datasets, which can be difficult to use due to their small size. Combining quick learning with robust features from small datasets is a difficult thing to do, as each of these three goals are difficult by themselves. We discovered a simple solution for this problem that is independent of the larger network structure.
What is next with this project?
We plan to apply this technology to other medical machine-learning problems that remain open. Our next problem focuses predominantly on kidney cancer.
What is next for you and how do you hope your work will impact society?
After I graduate, I plan on remaining in medical research, focusing mainly on bringing new medical machine-learning technologies into clinical practice. There is currently a large disconnect between medical research that has been developed and medical research that is used in practice, so I want to focus on helping that transition.
One passion of mine is to bring advanced technology and better medical care to populations with poor access to healthcare. Over three-fourths of heart disease deaths are in low to middle income countries. Better diagnosis would help decrease that, according to the World Health Organization. Focusing on generalization helps with that goal since expert analysis of one's health can be brought to patients more effectively using computers. This method can also perform well regardless of patient demographics.