Christopher P. Calderon
- Associate Research Professor
- CHEMICAL AND BIOLOGICAL ENGINEERING
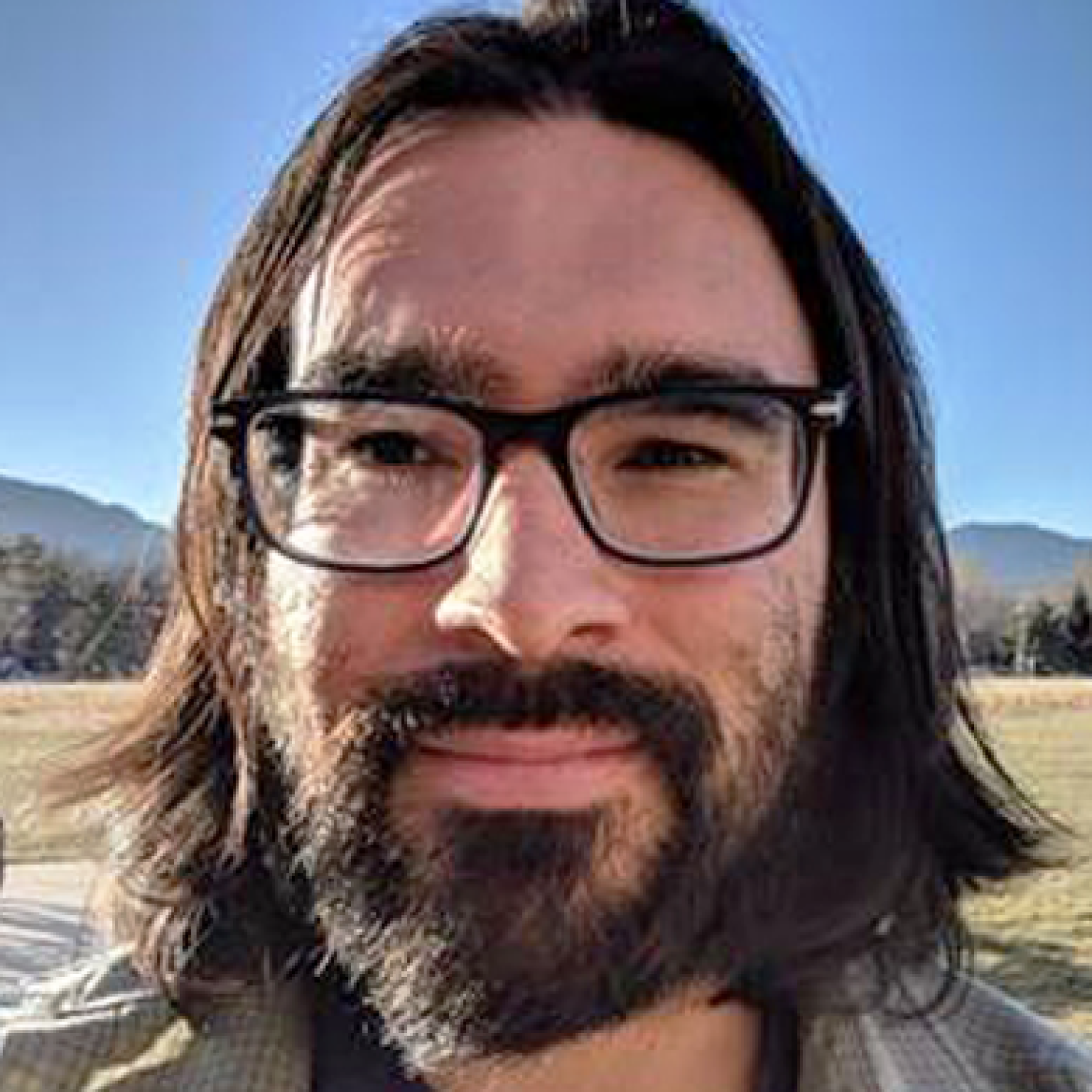
Education
B.S. Purdue University
Ph.D. Princeton University
Awards
- NIH "Nanobiology" Postdoctoral Fellow (2009)
- NSF VIGRE Fellow (2006)
- Gordon Wu Fellowship in Engineering from Princeton U. (2001)
- Lottes Memorial Award from Purdue U. (2001)
Selected Publications
- Calderon CP, Lewis AD, Randolph TW. Deep Convolutional Neural Network Analysis of Flow Imaging Microscopy Data to Classify Subvisible Particles in Protein Formulations. J. Pharm. Sci. (in press)
- Lee A, Tsekouras K, Calderon CP, Bustamante C, Presse S. Unraveling the Thousand Word Picture: An Introduction to Super-Resolution Data Analysis. Chem Rev. 2017;117(11):7276-7330. doi:10.1021/acs.chemrev.6b00729.
- Calderon CP. Motion Blur Filtering: A statistical approach for extracting confinement forces and diffusivity from a single blurred trajectory. Phys Rev E. 2016;93(5):53303. doi:10.1103/PhysRevE.93.053303.
- Calderon CP, Bloom K. Inferring Latent States and Refining Force Estimates via Hierarchical Dirichlet Process Modeling in Single Particle Tracking Experiments. PLoS One. 2015;10(9):e0137633. doi:10.1371/journal.pone.0137633.
- Calderon CP, Thompson MA, Casolari JM, Paffenroth RC, Moerner WE. Quantifying Transient 3D Dynamical Phenomena of Single mRNA Particles in Live Yeast Cell Measurements. J Phys Chem B. 2013;117(49):15701-15713. doi:10.1021/jp4064214.
- Calderon CP, Martinez JG, Carroll RJ, Sorensen DC. P-Splines Using Derivative Information. SIAM Multiscale Model Simul. 2010;8(4):1562. doi:10.1137/090768102.
- Calderon CP, Chen W-H, Lin K-J, Harris NC, Kiang C-H. Quantifying DNA Melting Transitions Using Single-Molecule Force Spectroscopy. J Phys Condens Matter. 2009;21(3):34114. doi:10.1088/0953-8984/21/3/034114.
- Calderon CP. On the Use of Local Diffusion Models for Path Ensemble Averaging in Potential of Mean Force Computations. J Chem Phys. 2007;126(8):84106. doi:10.1063/1.2567098.
- Calderon CP. Fitting Effective Diffusion Models to Data Associated with a “Glassy” Potential: Estimation, Classical Inference Procedures, and Some Heuristics. SIAM Multiscale Model Simul. 2007;6(2):656-687. doi:10.1137/050643647.
Research Interests
Dr. Calderon has been involved with developing new statistics and machine learning applications for uses in the basic physical and life sciences as well as industrial applications (industrial efforts at his company, Ursa Analytics, are focused on signal processing and target tracking). The common theme underlying the various applications are data-driven modeling and hypothesis testing associated with noisy, correlated data (images and time series data). He is particularly interested in interdisciplinary research collaboration aiming to leverage advances in machine learning and statistics to analyze images and signals recorded in nano to micro scale experimental systems. Applications have included time series analysis of single-molecule measurements to characterize biomolecules work in both in vivo and in vitro environments, supervised and unsupervised machine learning/image analysis applied to high-throughput devices, and analysis of noisy trace chemical detector signals in agricultural and military applications.
Research Focus Areas
Data-driven modeling of biological and chemical data, single-molecule experimental data analysis, time series modeling, machine learning applied to images and sensor measurements (supervised and unsupervised classification, detection, change point events analysis).