New software aims to speed and improve identification of DNA variations that lead to cancer
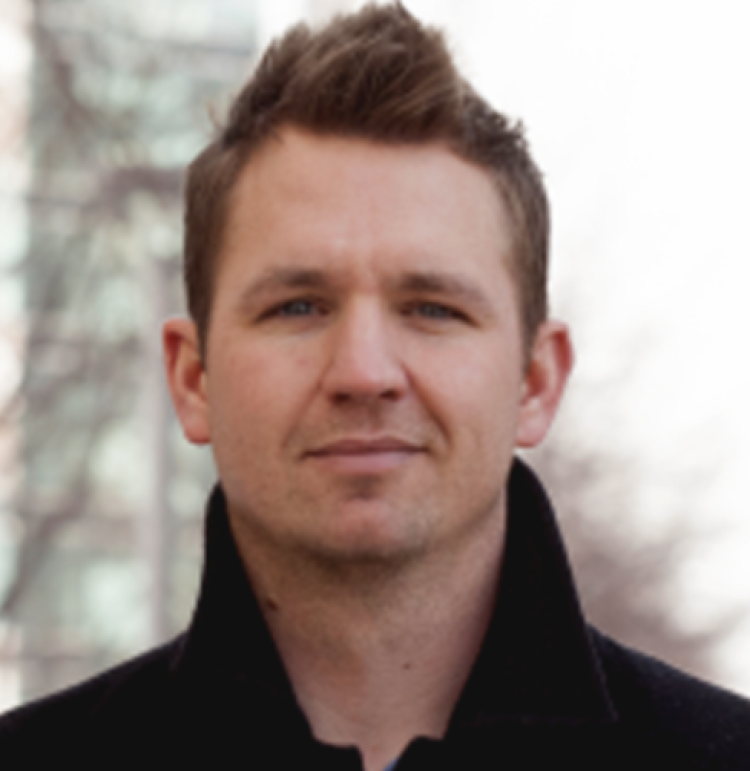
Layer
For years, researchers have been trying to quickly and accurately identify the parts of DNA that lead to genetic disorders like cancer. A new software tool from researchers at the University of Colorado Boulder could improve that process and lead to more tailored treatment and understanding of cancers from patient to patient.
“Understanding cancer requires identifying the genetic changes that forced a patient's healthy cells to grow uncontrollably,” said Assistant Professor Ryan Layer. “Unfortunately, any given tumor has thousands of these changes, and most are inherited – not mutated – or they have no effect at all. To identify variations that are problematic, we developed a technology that rapidly searches thousands of known genomes sets to identify the mutations seen only in the tumor.”
The work is part of ongoing research in Layer’s lab within the Department of Computer Science and the BioFrontiers Institute using algorithms to decipher very large genomic datasets. The new software – known as STIX – looks specifically at large structural variants that can lead to cancer. STIX uses a secondary analysis technique to search the raw data from thousands of samples, looking for any evidence supporting the existence of the variants in each specific tumor.
The process is described in a new paper in Nature Methods and aims to quickly characterize whether a particular genetic sequence is common or rare and potentially causing diseases like cancer in those specific tumor cells. The end goal is to provide patients with more tailored treatments based on findings from the sequences in their actual tumor compared to normal tissue. Ultimately, Layer said they want to provide that information in a way that is useable by anyone, anywhere.
“Hidden somewhere in the genome of a cancer patient's tumor are the mutations that encode the instructions for how the tumor started growing uncontrollably,” Layer said. “Unfortunately, the mutations that drive the tumor are mixed with instructions for all other aspects of human development and function – making it a complicated and time-consuming task to unravel.”
Murad Chowdhury, the first author on the paper and a staff scientist in Layer’s lab, said counting the occurrences of a sequence in a healthy population to help determine if it is a disease-driving mutation is not a new idea. However, the team’s approach extends the theory by including large genetic mutations, which require a fundamentally different approach to frequency estimation because they are harder to detect and characterize.
Chowdhury said the main challenge the team faced was computational – taking large data sets and reorganizing them to spend just one second searching the data for the needed information. Despite that, the method turned out to be an effective tool and could potentially be applied outside of medicine in the future.
“Our technique simultaneously reduces the data storage needs and improves the query speed so that analysis that would have taken months can go much faster,” Chowdhury said. “And by incorporating machine learning you can essentially use this background distribution for many potential future applications beyond cancer.”
Layer said a lot of work is done by researchers every year to analyze and catalogue information about tumors. The ultimate goal for his lab with tools like this is to make that investment worthwhile and useful.
“This tool is about improving access to data so users can get useful and accurate answers quickly,” he said. “Broad improvements to the ways we use this data allows us to re-use it in other instances and you wind up getting a lot more value out of the money you spend to get the data.”