Three CU Boulder aerospace grad students take off with NASA Fellowships
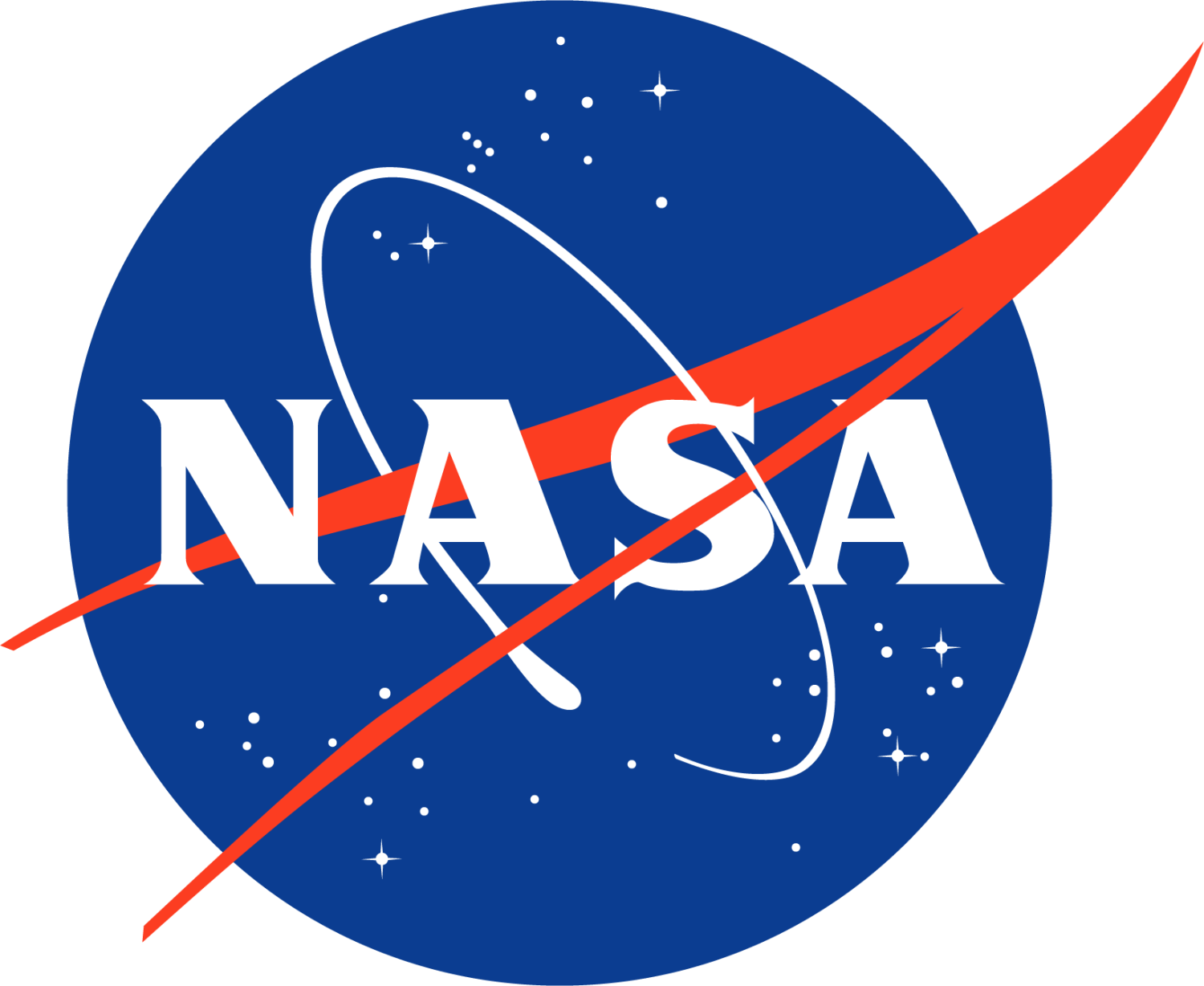
Three students in the Ann and H.J. Smead Department of Aerospace Engineering Sciences are being recognized with 2023 NASA Space Technology Graduate Research Opportunities (NSTGRO) fellowships.
The annual program sponsors graduate students who show significant potential to contribute to NASA’s goal of creating innovative new space technologies for our nation’s science, exploration and economic future.
Program recipients perform space technology research at their university campuses and at NASA Centers.
NSTGRO honorees receive research funding and are matched with a technically relevant NASA subject matter expert, who serves as a research collaborator.
The research collaborator functions as a conduit into the larger technical community corresponding to the student’s space technology research area.
Read below for more information about each honoree and their research.