Five aerospace students earn major NASA Fellowship
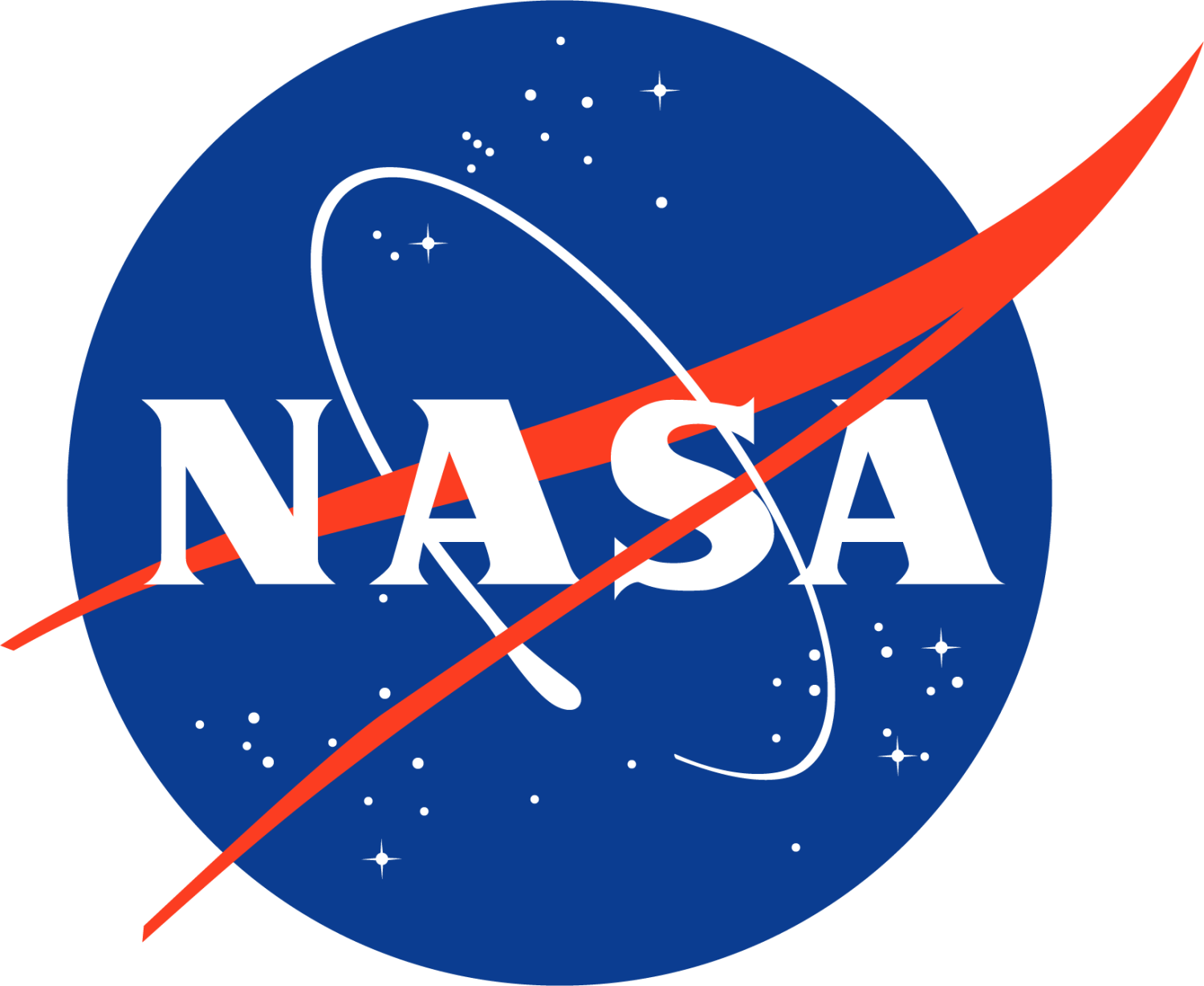
Five students in the Ann and H.J. Smead Department of Aerospace Engineering Sciences are being recognized with 2020 NASA Space Technology Graduate Research Opportunities (NSTGRO) fellowships.
The annual program sponsors U.S. citizen and permanent resident graduate students who show significant potential to contribute to NASA’s goal of creating innovative new space technologies for our nation’s science, exploration and economic future.
NASA Space Technology Graduate Researchers perform research at their respective campuses and at NASA Centers. Awards are made in the form of training grants to universities on behalf of individuals pursuing master’s or doctoral degrees, with the student's faculty advisor serving as the principal investigator.
In addition to their faculty advisor, each awarded student is matched with a technically relevant and community-engaged NASA Subject Matter Expert, who will serve as their research collaborator. The research collaborator will serve as the conduit into the larger technical community corresponding to the student’s research area.
Read below for more information about each honoree and their research.