Algorithms aren’t fair. Robin Burke wants to change that
Scroll through an app on your phone looking for a song, movie or holiday gift, and an algorithm quietly hums in the background, applying data it's gathered from you and other users to guess what you like.
But mounting research suggests these systems, known as recommender systems, can be biased in ways that leave out artists and other creators from underrepresented groups, reinforce stereotypes or foster polarization.
Armed with a new $930,000 grant from the National Science Foundation, CU Boulder Professor Robin Burke is working to change that.
“If a system takes the advantage that the winners in society already have and multiplies it, that increases inequality and means we have less diversity in art and music and movie making,” said Burke, chair of the Information Science Department at CU Boulder. “We have a lot to lose when recommender systems aren’t fair.”
Critics call out gender and racial bias
First developed in the 1990s, these complex machine-learning systems have become ubiquitous, using the digital footprints of what people clicked on, at what time and where to drive what apps like Netflix, Spotify, Amazon, Google News, TikTok and many others recommend to users. In the past decade researchers and activists have raised an array of concerns about the systems. One recent study found that a popular music recommendation algorithm was far more likely to recommend a male artist than a female artist, reinforcing an already biased music ecosystem in which only about a quarter of musicians in the Billboard 100 are women or gender minorities.
“If your stuff gets recommended, it gets sold and you make money. If it doesn’t you don’t. There are important real-world impacts here,” said Burke.
Another study found that Facebook showed different job ads to women than men, even when the qualifications were the same, potentially perpetuating gender bias in the workplace.
Meantime, Black creators have criticized TikTok algorithms for suppressing content from people of color.
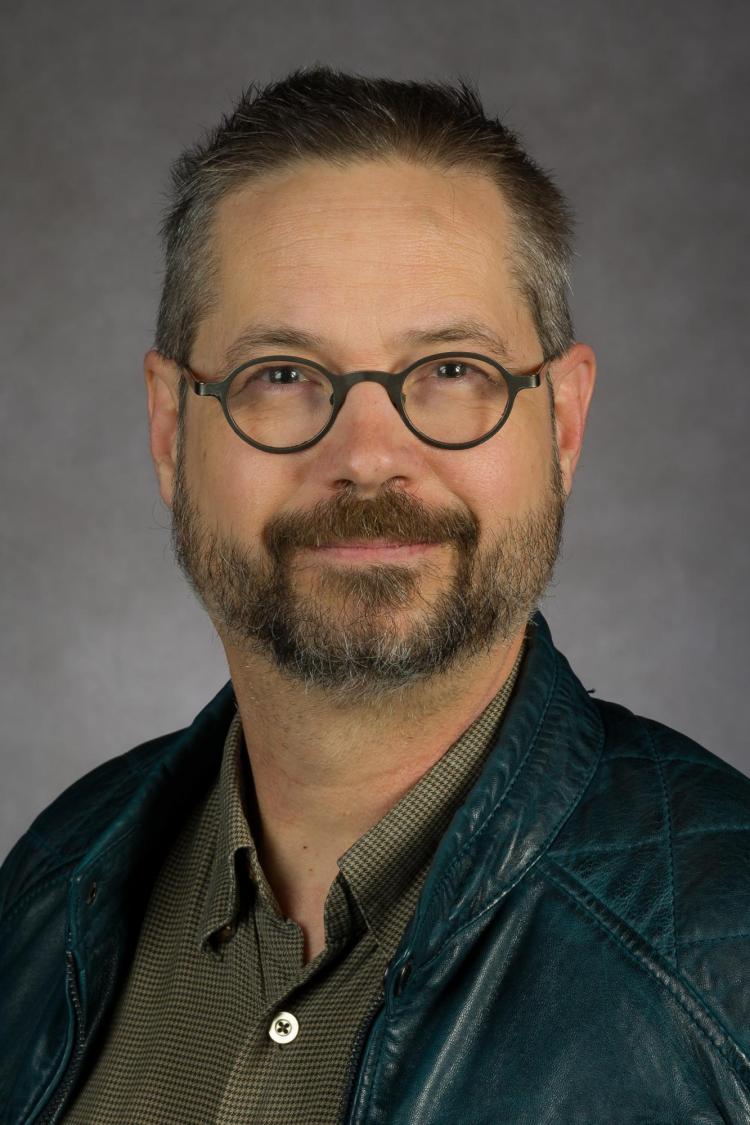
Professor Robin Burke
And numerous social media platforms have been under fire for making algorithmic recommendations that have spread misinformation or worsened political polarization.“If a system only shows us the news stories of one group of people, we begin to think that is the whole universe of news we need to pay attention to,” said Burke.
In the coming months, Burke, Associate Professor Amy Voida and colleagues at Tulane University will work alongside the nonprofit Kiva to develop a suite of tools companies and nonprofits across disparate industries can use to create their own customized “fairness-aware” systems (algorithms with a built-in notion of how to optimize fairness).
Key to the research, they said, is the realization that different stakeholders within an organization have different, and sometimes competing, objectives.
For instance, a platform owner may value profit-making, which might––in and of itself––lead an ill-crafted or unfair algorithm to show the most expensive product instead of the one that suits the user best.
An algorithm designed to assure that one underrepresented group of artists or musicians rises higher in a search engine might inadvertently end up making another group pop up less.
“Sometimes being fair to one group may mean being unfair to another group,” said co-principal investigator Nicholas Mattei, an assistant professor of computer science at Tulane University. “The big idea here is to create new systems and algorithms that are able to better balance these multiple and sometimes competing notions of fairness.”
Building a fairness-aware algorithm
In a unique academic-nonprofit partnership, Kiva––which enables users to provide microloans to underserved communities around the globe––will serve as co-principal investigator, providing data and the ability to test new algorithms in a live setting.
Researchers, with the help of students in the departments of Information Science and Computer Science, will conduct interviews with stakeholders to identify the organization’s fairness objectives, build them into a recommender system, test it in real-time with a small subset of users and report results.
No one-size-fits-all system will work for every organization, the researchers said.
But ultimately, they hope to provide a concrete set of open-source tools that both companies and nonprofits can use to build their own.
“We want to fill the gap between talking about fairness in machine learning and putting it into practice,” said Burke.