Projects
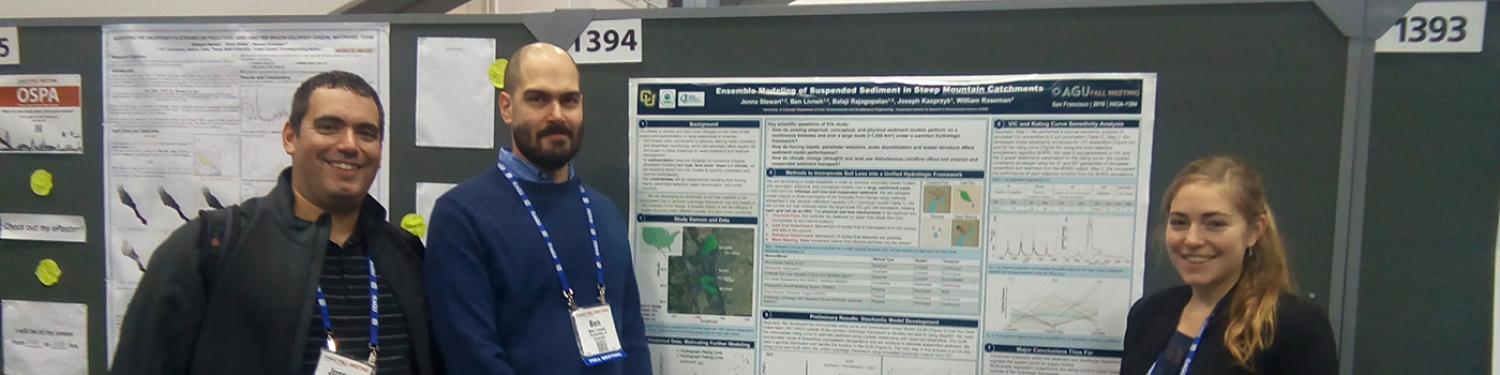
This page has a brief overview of our research approach as well as a list of our funded projects (see menu).
Why Many-Objective Analysis?
Policy analysis and federal planning has often been based on cost benefit analysis. The approach asks, do estimates of a proposed project’s benefits outweigh the expected costs? This has led to extensive use of single-objective optimization (that is, try to maximize a benefit function).
Why look beyond cost benefit analysis?
- Climate change and population growth complicate our traditional strategies for water supply. How can we project the proportion of water use needed for electricity generation, for example? USGS water withdrawal data shows that a larger percentage of water is used for these purposes now than in 1950. Will climate change cause this propotion to increase?
- Under these conditions, it is difficult or impossible to commensurate all decision makers’ preferences into a single objective function. Using the example above, in extreme droughts, the preferences of electricity generators may strongly conflict with municipal supply, or there may not be enough water to meet environmental low flow conditions. This motivates a method to discover tradeoffs, both between different use categories and between different performance objectives (such as maintaining supply reliability).
- Innovative solutions to these problems will likely require portfolios of management strategies. The portfolios could include improved management of existing supplies (new guide curves), new supplies such as desalination and water reuse, and transfers in water markets. Simulations of water supply portfolios are highly non-linear, with strong “threshold” effects based on decision maker actions. This motivates a method that can fully integrate complicated simulations into optimization and decision support.
Our group’s research advances a many-objective approach to water planning and management. We use advanced evolutionary search tools to develop tradeoffs for problem formulations that can explicitly show how conflicting objectives compare. For example, what is the cost of adding an additional unit of performance in a supply system? What is the highest level of environmental flow that can be achieved at every level of cost and reliability?
An important aspect of the approach is that trusted, full-complexity simulations are integrated into the search algorithm. It allows a rich level of detail in understanding the physical system, with all nonlinearities preserved when mapping decision maker actions to outcomes.
The search provides a number of diverse alternative designs for the system. Instead of trying to model decision makers’ preferences before the model runs are complete, we use an a posteriori approach that gives them a wealth of information to inform their selection. This is supported by interactive visualizations that provide an immersive experience where analysts can understand the implication of design decisions.