F-formations
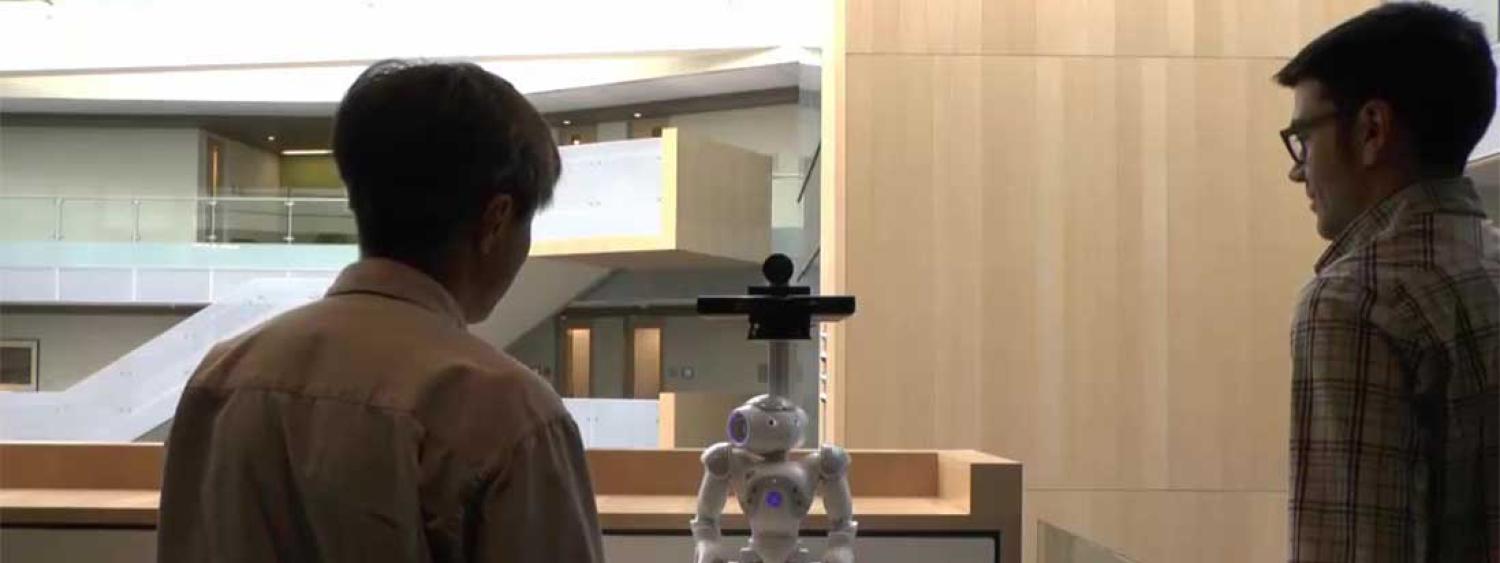
Associated Researchers
Publications
Hooman Hedayati, Annika Muehlbradt, Daniel J. Szafir and Sean Andrist. 2020. REFORM: Recognizing F-formations for Social Robots. (to appear) In: Proceedings of the 2020 IEEE/RSJ International Conference on Intelligent Robots and Systems (IROS 2020). (Las Vegas, Nevada–October 25-29, 2020).
Hooman Hedayati, Daniel Szafir, and James Kennedy. 2020. "Comparing F-Formations Between Humans and On-Screen Agents". In: Extended Abstracts of the 2020 CHI Conference on Human Factors in Computing Systems (CHI EA ’20). (Honolulu, Hawaii (virtual) – April 25-30, 2020).
Hooman Hedayati, Daniel Szafir, and Sean Andrist. 2019. "Recognizing F-Formations in the Open World". In: 2019 14th ACM/IEEE International Conference on Human-Robot Interaction (HRI '19). (Daegu, South Korea – March 11-14, 2019).