ATLAS researchers' algorithm helps robots detect everyone in social gatherings
To effectively participate in a group discussion, it's important to be able to identify who is present and direct your attention accordingly. For most people, this is not hard, but designing robots able to do the same thing is quite challenging. While robots are equipped with sensors for detecting the number of people in a group, they are not always accurate and, to date, there has been little research into how robots can confirm their assumptions and correct any errors they may have made.
However, last year, two researchers in ATLAS Institute's IRON Lab* developed a solution to this problem that is described in a paper published in the March Proceedings of the International Conference on Human-Robot Interaction (HRI '22). The authors, Hooman Hedayati (PhD computer science '20) and Daniel Szafir, assistant professor of computer science at UNC Chapel Hill and the former director of the ATLAS IRON Lab, proposed a method to overcome situations when conversational group (F-formation) detection algorithms fail.
By studying different conversational group data sets, the researchers observed that relative to the size of a conversation group, people tend to stand in predictable locations relative to each other. Hedayati and Szafir then developed a system for identifying high probability regions where people are likely to stand in a group relative to a single participant. Using that system, the robot can reason when another person in the conversation hasn't been detected and correct their error.
The first model estimates the true size of a conversational group, where only some participants were detected. The second model predicts the locations where any undetected participants are likely to be standing. Together, these models may improve detection algorithms and a robot's ability to detect members of a group and participate more seamlessly in a conversation.
*Following Szafir's departure last summer, the ATLAS IRON Lab was closed.
Publication
Hooman Hedayati and Daniel Szafir. 2022. Predicting Positions of People in Human-Robot Conversational Groups. In Proceedings of the 2022 ACM/IEEE International Conference on Human-Robot Interaction (HRI '22). IEEE Press, 402–411 (March 7-10, 2022—virtual, originally Hokkaido, Japan).
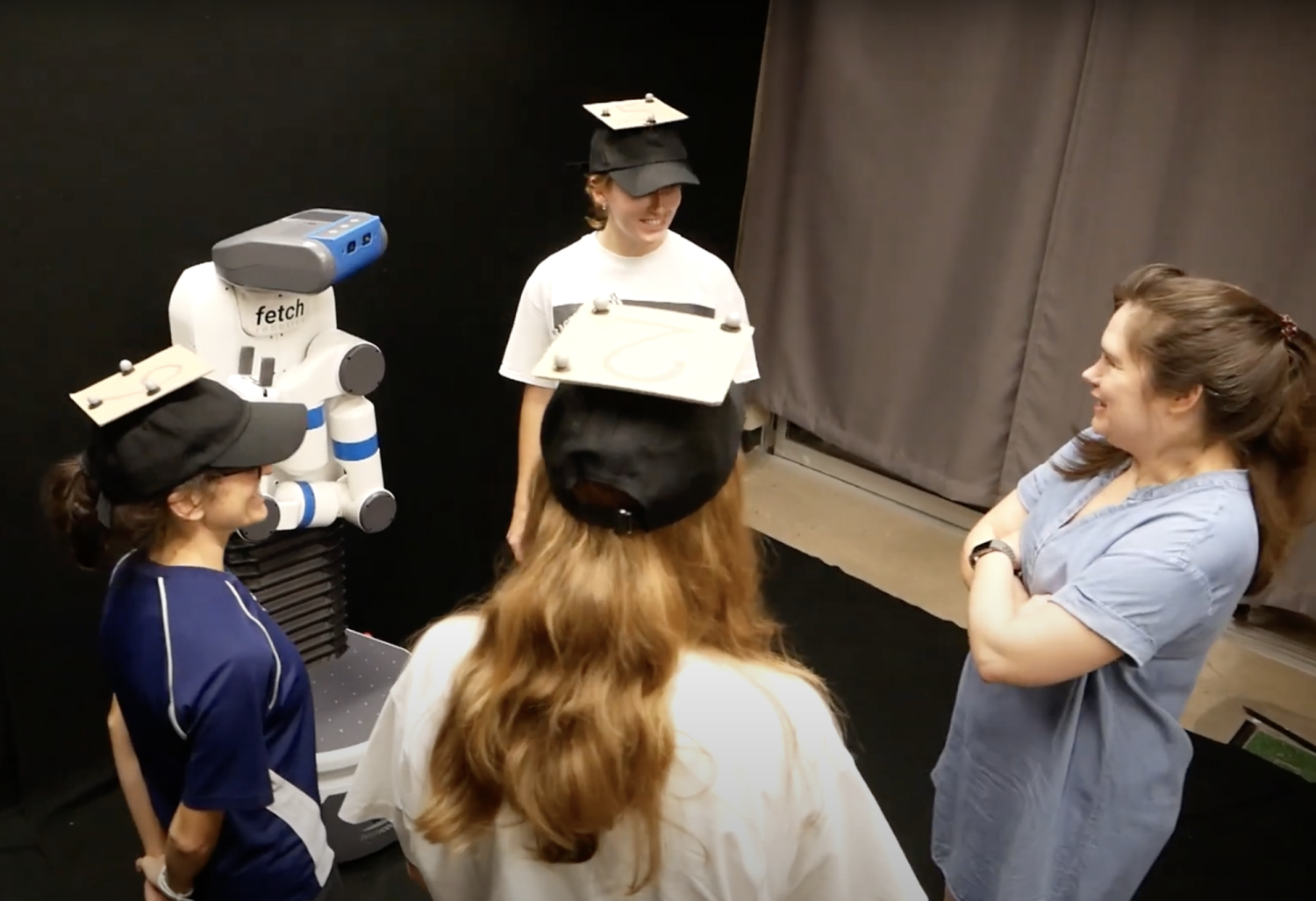