MISA Seminar (May 3, 2022): "Machine Learning Prediction of Global Ionospheric TEC and High-latitude ROTI Maps"
Machine Intelligence for Space Applications (MISA) Seminar Series:
Hosted by: CU's Space Weather Technology, Research, and Education Center (SWx TREC)
Presented by: Lei Liu (co-authors Y. Jade Morton, Yunxiang Liu)
Tuesday, May 3rd 2022, 2:00pm – 3:00pm (MT)
Zoom Recording: https://vimeo.com/710534362
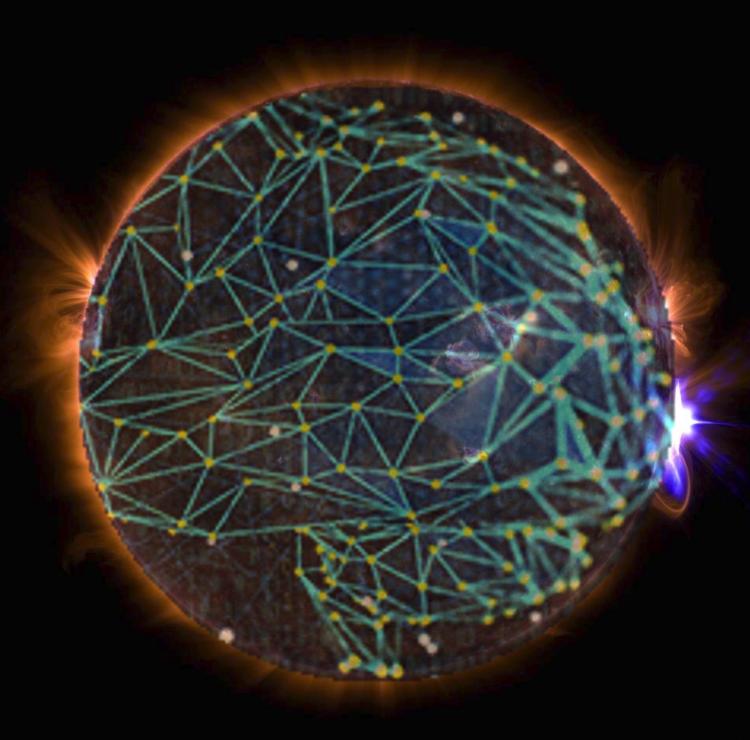
In this talk, we will present an image-based convolutional long short-term memory (convLSTM) ML algorithm to predict global TEC and storm-time high-latitude rate of TEC index (ROTI) maps. Results show that:
- The convLSTM-based ML algorithm that combines the L1 loss function and residual prediction strategy shows satisfactory performance in prediction of global TEC maps with up to 24 hours of lead time at a 1-hour interval when compared to other combinations.
- The prediction results for the irregulates-related ROTI maps outperforms the state-of-the-art model with a lead time of up to 60 minutes because of the utilization of the convLSTM-based algorithm and a custom-designed loss function.
Categories: Events