Research
Prediction serves as the ultimate test of our scientific understanding of geophysical systems. Accurate forecasting of near-Earth space environmental conditions is critical to radio communication, navigation, positioning, and satellite tracking. Effective numerical prediction of the region’s conditions allows us to better protect important space assets and related systems in the event of natural hazards.
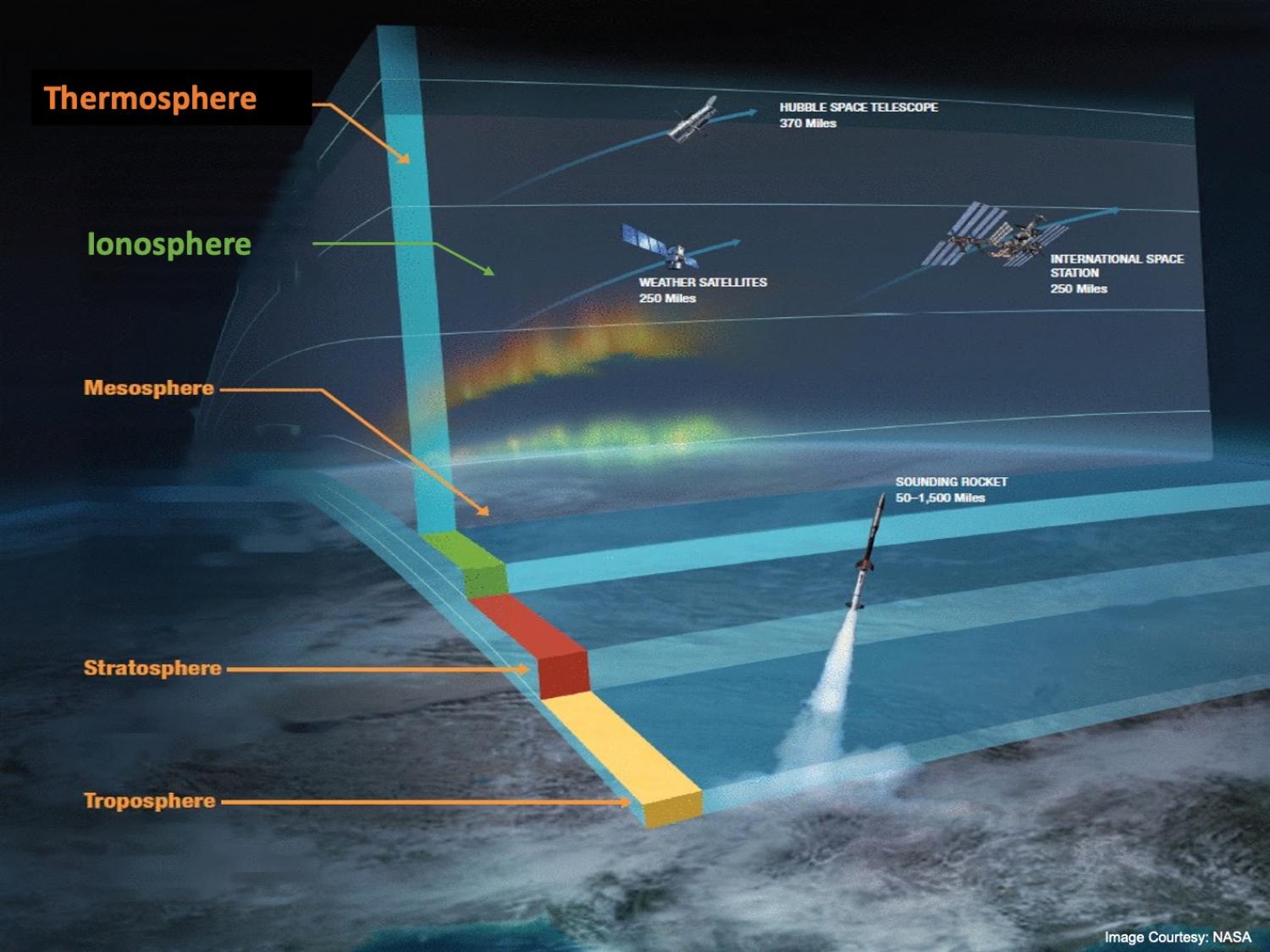
Geospace Data Science Lab aims to advance the science and engineering of forecasting, as applied to the Earth’s atmosphere from the ground to near-Earth space environments, while developing fundamental understanding of the predictability of a coupled system of the whole atmosphere, ionosphere, and magnetosphere. Prediction of constantly changing environmental conditions, affected by both space and terrestrial weather, requires a systematic integration of observations with a first-principles models using data assimilation. Data assimilation reduces uncertainties in initial conditions and drivers, extending the predictive capability of numerical models of near-Earth space environments. The data assimilation and ensemble-based probabilistic modeling framework being developed can be used for designing of future missions and targeting of observations to maximize scientific returns of observing systems. Geospace Data Science Lab also focuses on methodological problems, including the development of scalable data assimilation methods for high-dimensional problems, inversion and machine learning techniques to extract relevant geophysical information from large volumes of heterogeneous remote sensing and in-situ sensor data.